Arnak Poghosyan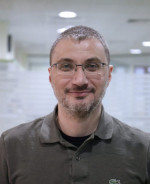
Arnak Poghosyan, Ashot Harutyunyan, Naira Grigoryan, and Nicholas Kushmerick
VMware, Inc.
Abstract:
Identification of a problem fingerprint or incident in a data center is of crucial importance for the system administrators. Automated discovery of such important patterns in cloud environments is recently gaining a lot of popularity for effective and efficient root cause analysis of business-critical is-sues. A problem incident is a group of alerts with sufficient historical evidence in reoccurrence and similarity. Presumably, all known incidents should be stored in user’s knowledge base together with available annotations regarding the problem description and its possible resolutions. The knowledge base of incidents with enough coverage of system’s possible failures is an invaluable asset for any system administrator. It will help to detect and isolate a problem, accelerate its remediation. In many cases it will also help to anticipate upcoming performance degradations before they impact the system. This classical approach totally relies on authentic alert definitions which mostly require expert knowledge regarding the environment peculiarities which makes impossible automation of the root cause analysis of unknown and very complex systems. In this paper, we consider essentially different approach that bypasses the alert definition and management process. Application of rule-learning algorithms to system indicators defines appropriate incidents in terms of rules with enough statistical evidence. We consider different possibilities both with labeled and unlabeled metric spaces. The labels can be derived from users’ feedbacks on system failures or performance degradations. In case of unlabeled metric space, outlier detection procedures can be applied for data labeling. |
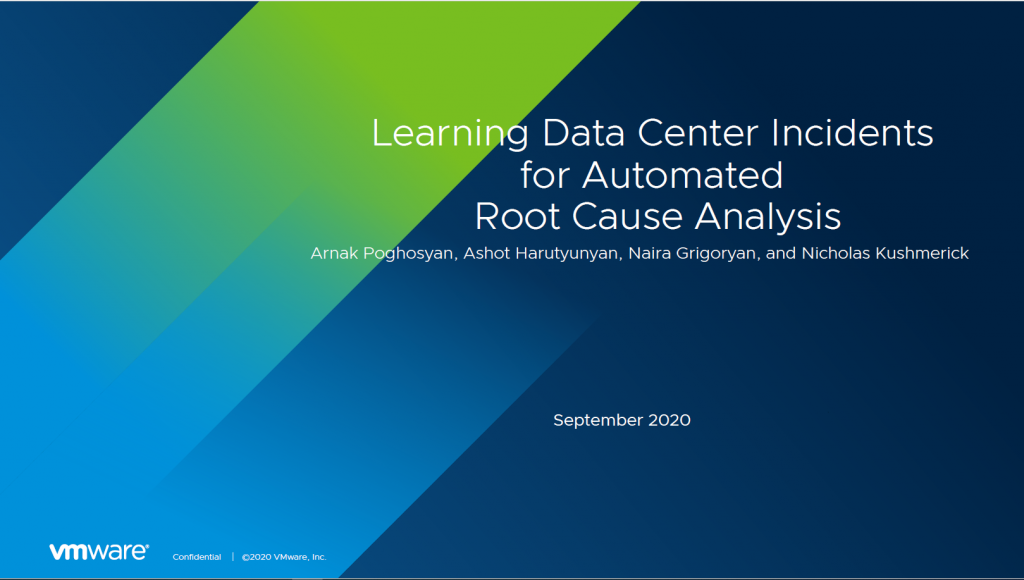
Discussion Room: Learning Data Center Incidents for Automated Root Cause Analysis